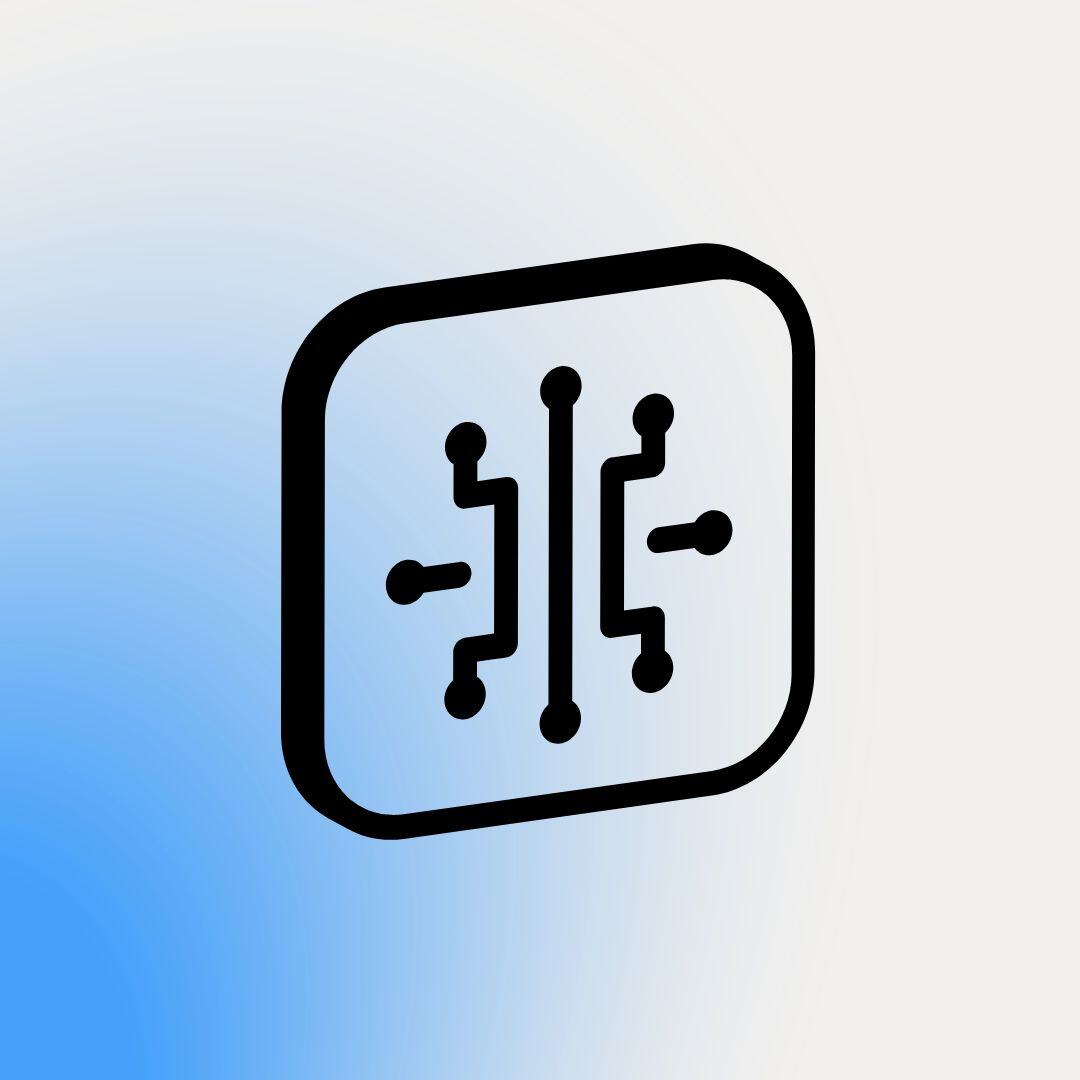
AWS Generative AI
Securely Build With Generative AI on AWS
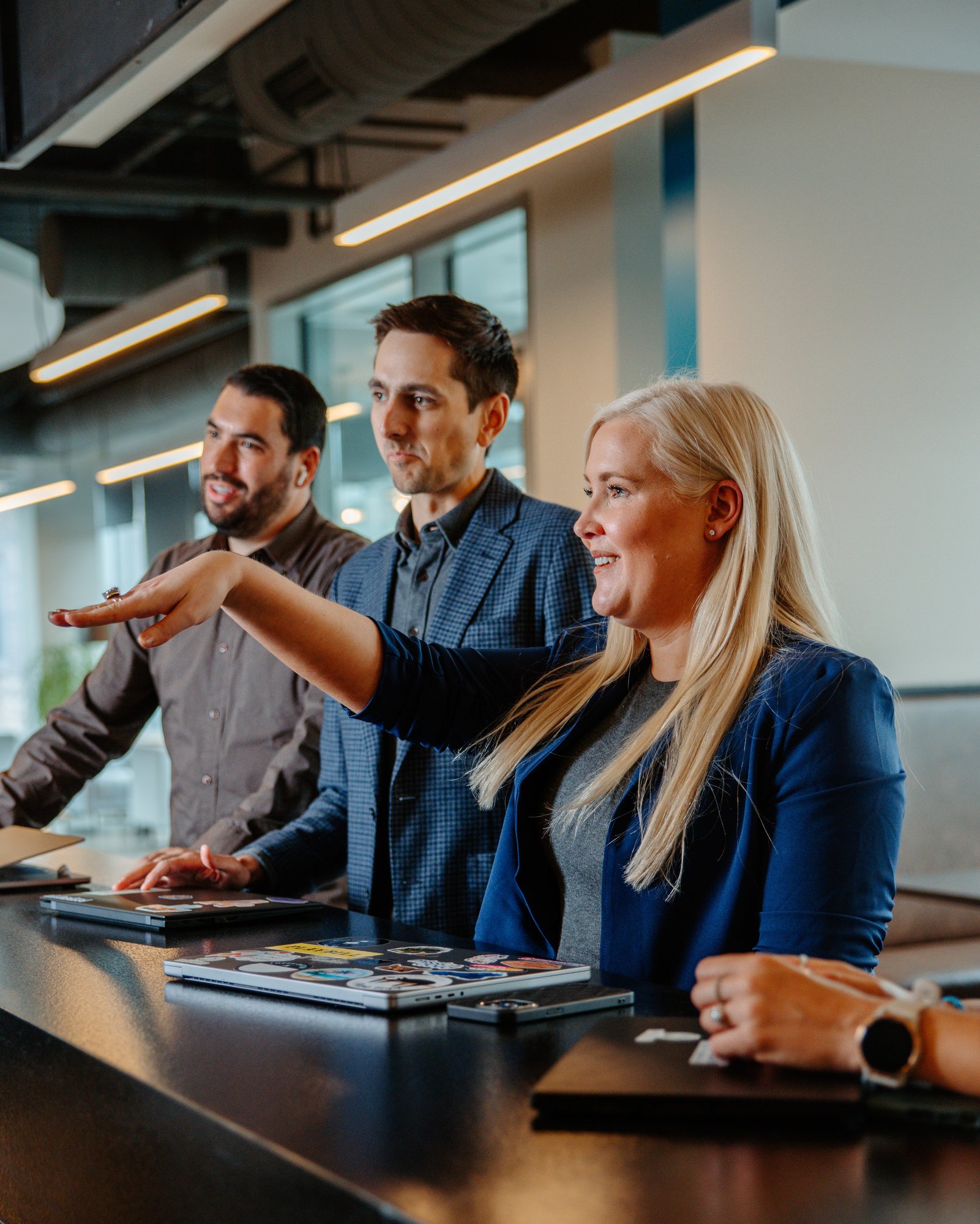
Transform Your Business with Generative AI
Explore the vast potential of generative AI on AWS to generate original content and concepts for your business, revolutionize your applications, create novel customer experiences, improve productivity and transform your business operations. Amazon has launched Bedrock and Foundation Models accessible through SageMaker Jumpstart allowing you to use your internal data to train your models while keeping your data private.
Mission can support you with in-depth experience and technical expertise whether you’re just getting started or need to scale your pre-existing model’s infrastructure.
Customer Success Story: Paynela
AI-Driven Patient Financial Assistance
Paynela, a Puerto Rico-based healthcare financing innovator, partnered with Mission to revolutionize patient financial assistance through cutting-edge solutions powered by AWS.
Through Mission's expertise in AWS technologies and AI integration, Paynela now uses gen AI to analyze complex healthcare data, resulting in deeper insights and expanded patient support capabilities, making healthcare more accessible and helping patients manage out-of-pocket medical expenses with dignity and ease.
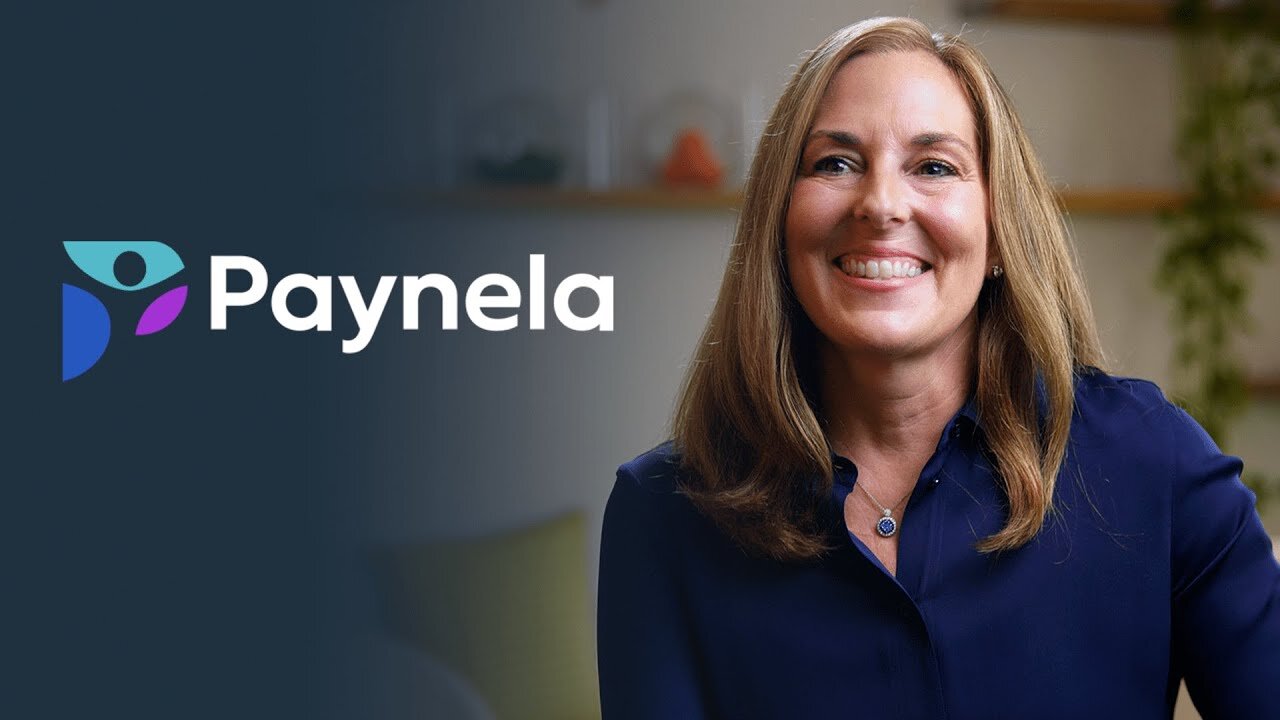
Accelerate Your Use Case
-
Customer Service
Resolve customer requests and answer common questions with AI-powered knowledge bases and chatbots, call transcription, and conversation summaries for helping to resolve cases.
-
Document and Image Analysis
Extract key takeaways or synthesize documents and images without requiring the presence of a human expert. Transform legal, financial, and medical paperwork into actionable data.
-
Media Translation
Create automated dubbing and translation pipelines for your content generation. Cater to an international audience with fast and accurate video, audio, and text translation.
-
Coding Assistance
Turn to generative AI tools such as Amazon CodeWhisperer for coding, particularly for initial prototype work, integrating an unfamiliar library, or helping with debugging.
-
Personalized Content Creation
Generate original content from your data, such as product documentation or personalized marketing campaigns, with messaging tuned to fit your brand, channel, and audience.
-
Image Generation
Leverage the creativity of large language models and tools like Stable Diffusion to generate novel images and graphics for your marketing.
Explore the vast potential of generative AI on AWS to generate original content and concepts for your business, revolutionize your applications, create novel customer experiences, improve productivity, and transform your business operations.
Get Help Building and Scaling
Whether you’re scaling your model for production or starting from scratch, we meet you where you are on your journey and help you get your solution launched.
Our Process
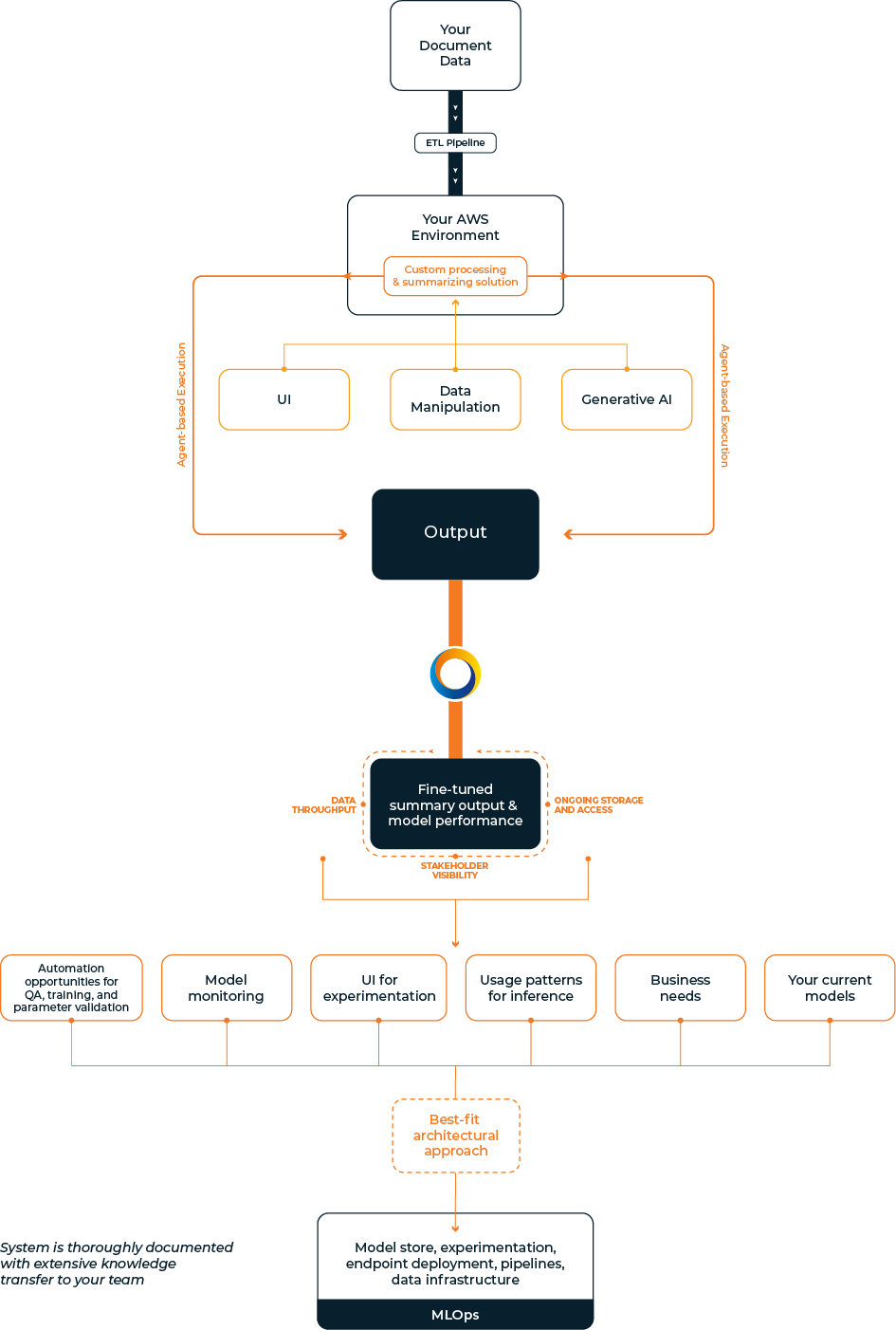
Tools We Use to Build and Scale Generative AI Applications
“We were immediately impressed with Mission’s expertise both on AWS and with generative AI. Working with Mission has not only provided much needed AWS expertise to ensure our business is secure, reliably online, and optimized, but also to build out a bleeding-edge Machine Learning pipeline on top of AWS cognitive services. Partnering with Mission will enable us to achieve a long-held goal for MagellanTV, which is to massively expand the reach of our documentary platform and strengthen our position as a truly global company.”
I’m interested in generative AI on AWS. How do I know if it’s a good fit for my business?
If you don’t have a clear use case in mind, it can be useful to start with a business objective and work backwards from there. This is how we typically structure engagements, and we’ve found it to be a powerful tool for identifying opportunities and ranking their value. To be perfectly transparent, not every problem is a good candidate for gen AI capabilities, but when you have one, the upside can be huge.
I’m concerned about the privacy of my data. How can I ensure I’m not risking sensitive information when working with a model?
Consider the architecture you’re working with and the nature of the guarantees you need. Some model makers will offer that no data is stored once you are paying for an Enterprise plan, for instance. But for some data, you may find that the notion of sending it over the public internet is untenable. If that’s your situation, consider services like Amazon Bedrock, which allow you to keep your data and access the model entirely within AWS infrastructure and therefore maintain the associated security and privacy guarantees.
I’ve heard generative AI can be expensive. How do I make sure I’m budgeting appropriately?
We can perform a Total Cost of Ownership analysis, to help you understand your infrastructure costs once your solution is up and running. But the key to developing a cost-efficient solution is to use the proper techniques for your problem. For example, retrieval-augmented generation can vastly reduce the size of prompts you need to use. You may also want to consider model fine-tuning, quantizing your model, and training it to your specific use case to avoid the overhead of running a large model if you don’t need that level of generalized power.
My solution sometimes responds with inaccurate or made-up answers. How do you handle that?
Yes, without training or providing your model with the exact information you're querying through a technique like retrieval-augmented generation, you may find your solution “hallucinates” an answer or doesn’t alert you that it doesn’t have the specific answer. How a model reacts in each case depends on how it was developed, the specifics of your prompts, and the surrounding solution. As part of our methodology when building a solution, we tune performance until you’ve reached an acceptable error rate, ideally zero. And we may also suggest developing with a different model if you need highly-specific outputs.
It seems like there’s a lot of hype in this space. Will a generative AI solution actually make a difference for my business?
The honest answer is: not always. While models are highly flexible problem solvers and their capabilities are continuously evolving, not every problem is efficiently solved with a large language model as part of the implementation. With that said, when you have a good fit for this technology, the upside can be tremendous. In general, the best use cases involve enhancing your existing capabilities, removing human intervention or manual work from a process, accentuating customer experience, or quickly generating outputs for prototypes of work like code, text, or images.
I’ve got a great use case, but my team doesn’t have much experience with this technology. How should we get started?
Experimenting with prompts can be a great way to start early prototyping. AWS SageMaker and SageMaker Studio also provide a platform for trying different models, quickly launching infrastructure, and working with an interactive notebook to try solutions and integrate your data. This is a great starting place for building a proof of concept. But if that sounds too daunting or you’ve reached your technical limits with that approach, consider engaging with a partner like Mission. We’ll get up to speed on the state of your current work, evaluate your objectives, and help you build a roadmap for how to get there. If you want our help building the implementation, infrastructure, and algorithms, we can do that too!
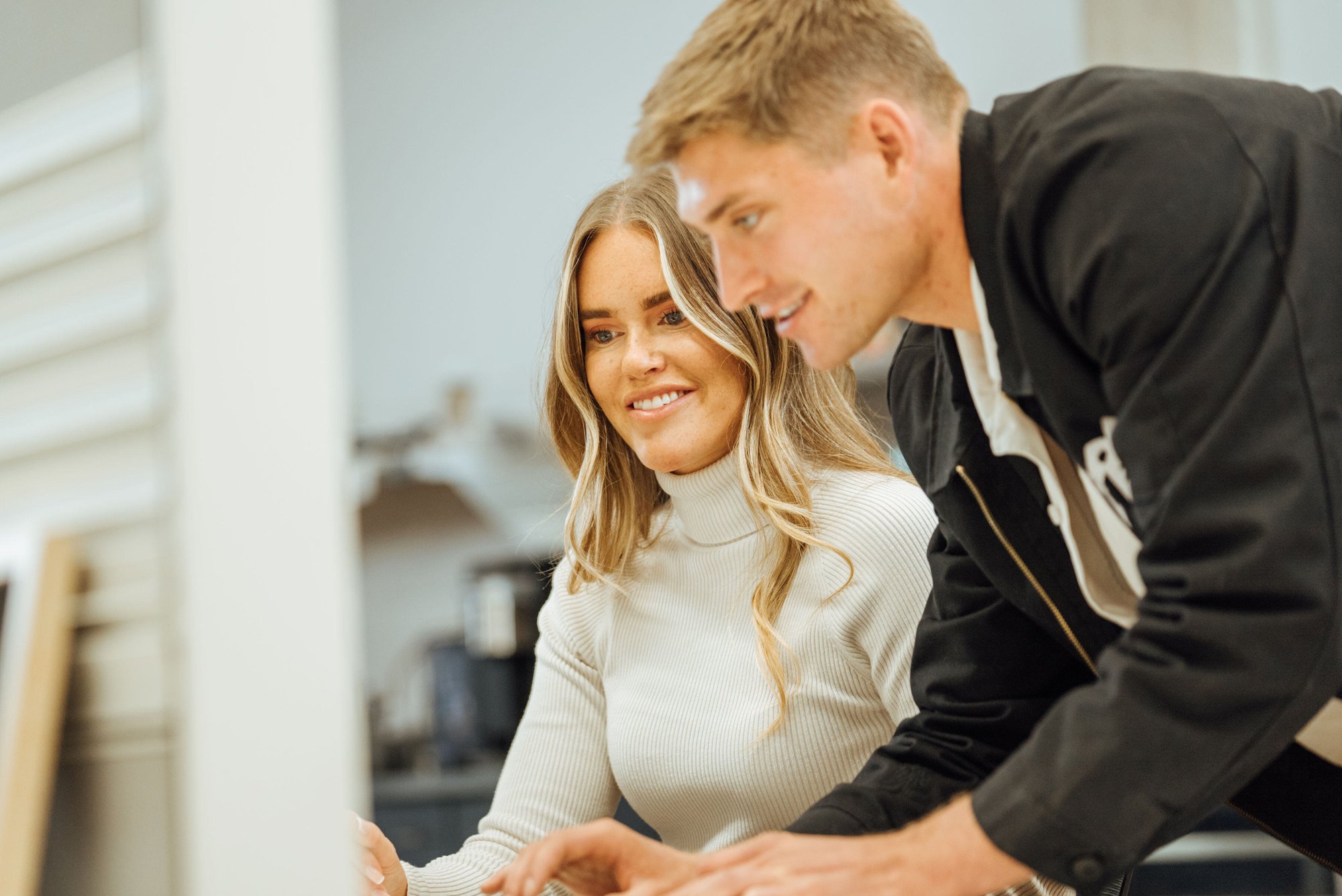
Get in touch
Succeed With Generative AI on AWS
Work with a genAI partner who understands machine learning on AWS, your goals and the agile processes required to bring value to your business faster.
Find In-Depth Guides, Articles, AWS Best Practices and More
Continue your cloud journey by learning from our cloud experts. We share insights and best practices on everything from app development and migrations to cost optimization and generative AI.
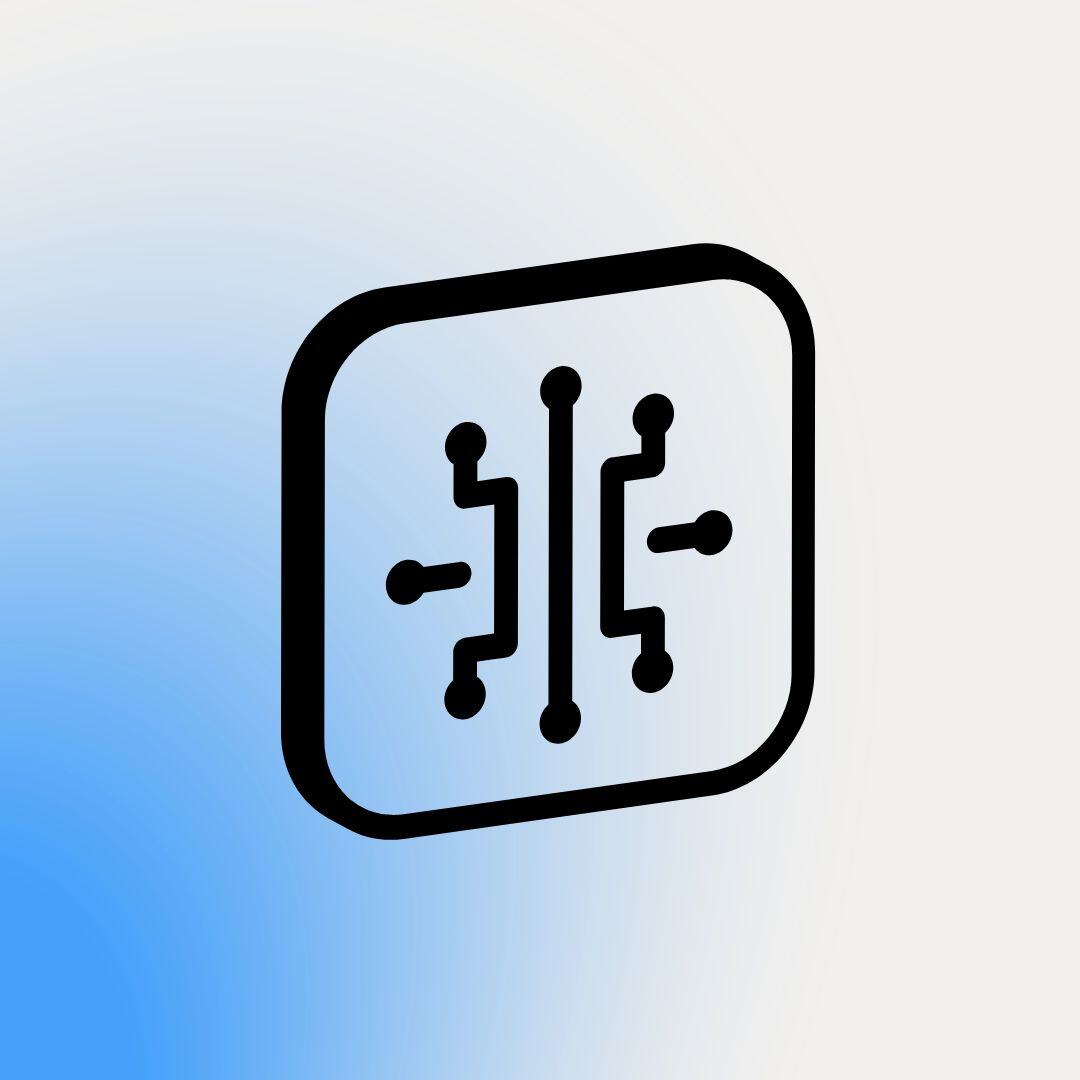

How to Measure Your AI Maturity
Categories:
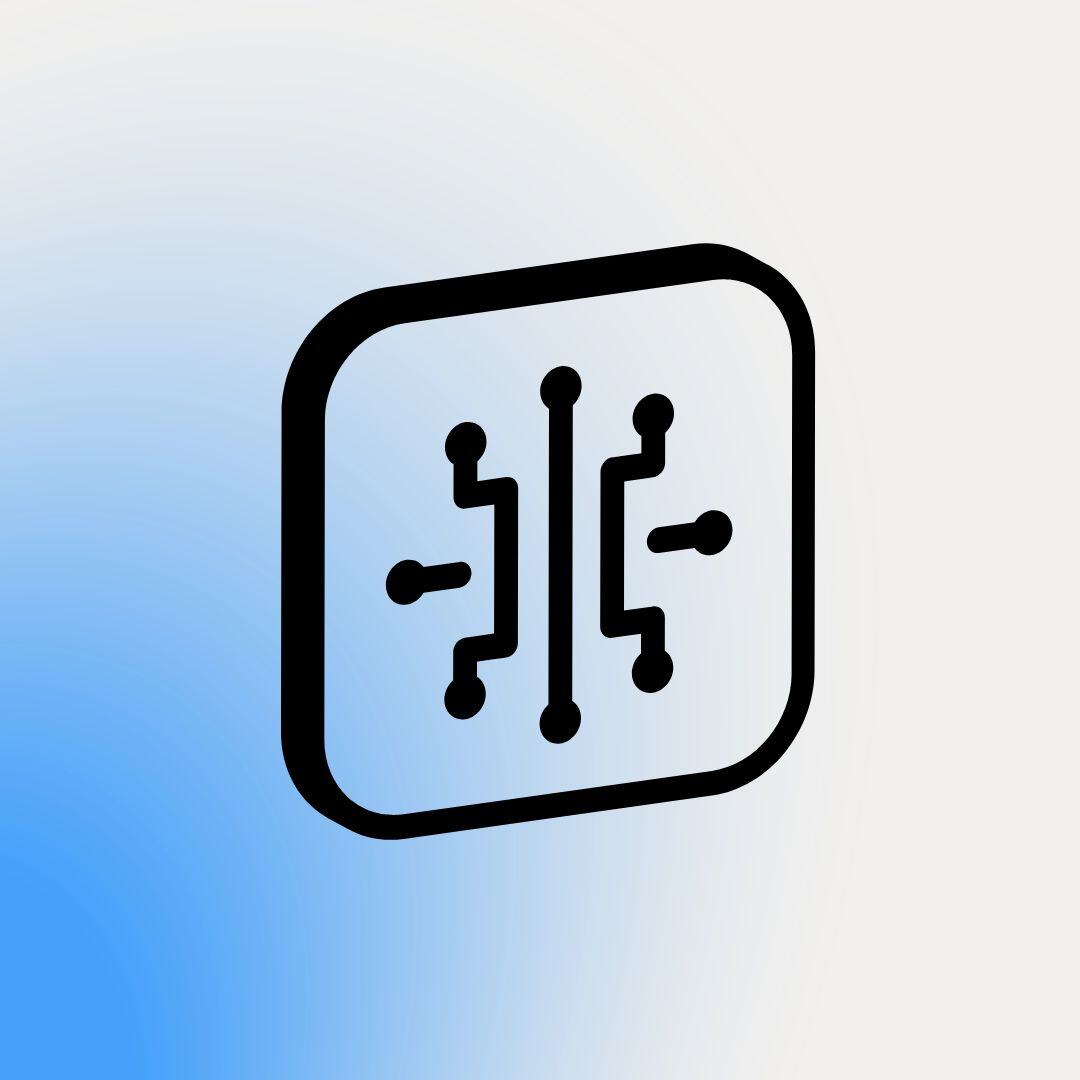