Blog
10 Use Cases for Machine Learning in Financial Services
From credit card transactions to stock prices, data science plays a pivotal role in the economy and the financial services that support it. One of the biggest problems with data can be the overwhelming amount that businesses need to analyze. Fortunately, machine learning in financial services is helping firms quickly analyze data and apply insights faster than humans can on their own.
Artificial intelligence and machine learning in financial services are already creating value and continue to evolve at a rapid pace. According to a CFA Institute survey, new analytical methods — including artificial intelligence and machine learning — are expected to transform the industry’s job roles in the next five to 10 years.
Financial service providers have an opportunity to leverage machine learning to create efficiencies, control costs and improve performance. Learn about why financial firms like yours are embracing machine learning, the challenges of implementation and 10 ways your firm can get started.
What’s the Need for Machine Learning in Finance?
The financial services industry has been particularly receptive to machine learning because the industry operates in real time and generates vast amounts of data. Financial firms need a way to analyze data quickly with less human involvement.
Traditional methods, such as manual analysis or rules-based systems, are insufficient for quickly sorting and sifting through a large amount of data. Machine learning allows you to leverage powerful algorithms that can process data from multiple data sources and decipher patterns, and create systems that can predict future outcomes. As this technology continues to evolve, financial institutions that don’t embrace machine learning will struggle to keep up with those institutions that do.
Using machine learning in the finance industry isn’t just about efficiency and savings. It’s also about creating value for customers, including tailored recommendations that help them reach their goals. Machine learning, along with effective MLOps practices, can help you better understand your customers, their needs and how you can improve their experience.
Challenges of Using Machine Learning in Financial Services
Machine learning and financial services both rely on large sets of highly complex data. Managing that complexity isn’t easy. Here are three common challenges of machine learning.
Data Bias
A challenge facing any modern use of data for predictive insights is bias. This is true of machine learning and artificial intelligence in financial services, too. Such bias can be the fault of the algorithm, but even the best algorithm is only as good as the data it’s being fed. For example, if a model is trained on customer data from specific ZIP codes, it may mistakenly discriminate against people that live in other areas, creating disparities and inadvertent discrimination in credit access, financial assistance or other important services.
Another way data bias creeps into machine learning models is through human bias. For example, if the people who are labeling data for a machine learning model have personal biases, these biases can be reflected in the model. It is important to understand the biases in your data to create comprehensive data sets and different models to serve each use case.
Complexity and Compliance
Financial data is often complex, making it difficult to develop effective machine-learning models that meet the industry’s needs. Additionally, the financial sector is heavily regulated and scrutinized. Any machine learning model you choose must be considered in terms of compliance with today’s regulations and with an eye toward anticipated legal, regulatory and privacy challenges. With all of the regulations and potential for your system to be audited, it is critical to develop an MLOps strategy that keeps track of your data sets and model outputs for regulators to review.
Hiring and Retaining Quality Employees
There's a shortage of talent with the necessary skills to build and implement effective machine-learning models across industries, including financial services. This shortage means that organizations must pay premium wages or outsource the work to a third party, such as an AWS data analytics and machine learning partner.
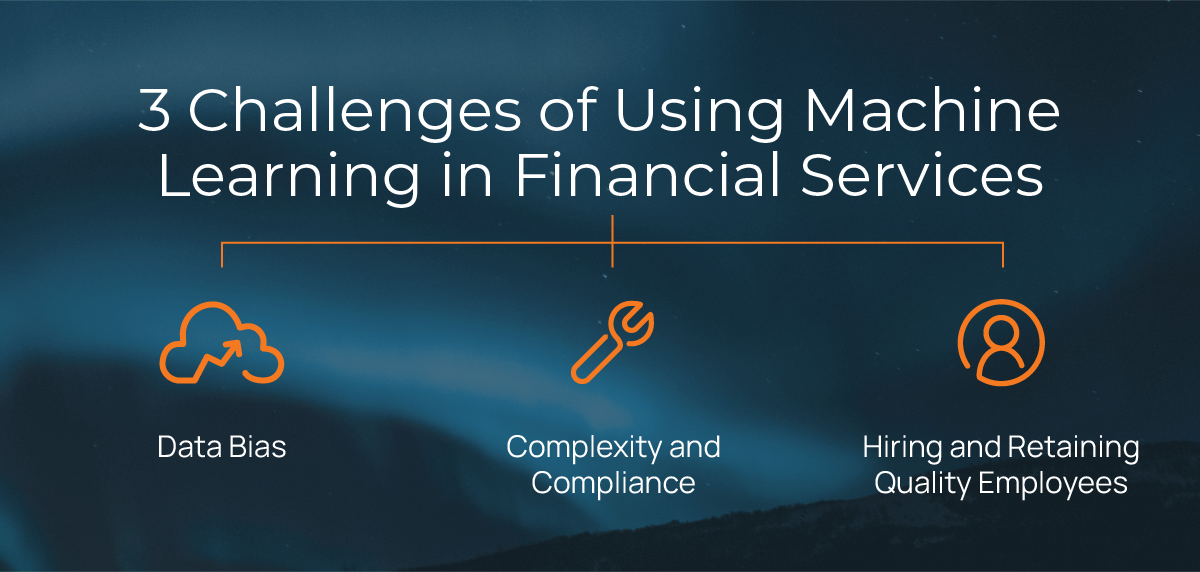
10 Ways Machine Learning Is Used in Financial Services
Machine learning is an evolving field but is already proving its value to the financial services industry. Here are 10 ways your organization can begin using it.
Fraud Detection
Traditional methods of fraud detection involve rules-based systems that flag suspicious transactions based on predefined criteria. However, these systems are inflexible, need frequent updating and result in false positives. Machine learning, by contrast, can analyze data quickly and with greater accuracy. It identifies patterns and anomalies to predict potentially fraudulent activities.
For example, Amazon Web Services (AWS) offers Amazon Fraud Detector, which uses machine learning algorithms to analyze transaction data and generate fraud risk scores in real time.
Because fraud is a constant threat, your methods of detection need to evolve as bad actors become more sophisticated in their fraud attempts. Machine learning algorithms help prevent fraud and reduce financial, operational and reputational risks.
Customer Service
Artificial intelligence and machine learning in financial services have made it easier for institutions to provide customer service. For example, by combining machine learning techniques with natural language processing (NLP), another subset of AI, chatbots and virtual assistants can easily understand and respond to people’s queries and requests.
These tools free up customer service representatives to handle more complex issues. They also offer another source of data that can be used for personalized recommendations based on a customer's history and preferences.
Risk Assessment
Artificial intelligence and machine learning techniques can expedite risk assessment activities while improving quality. Loan underwriting, for example, benefits from using machine learning to analyze data for patterns or inconsistencies that might indicate fraudulent behavior, potential loan defaults or other risk.
You can use services such as AWS SageMaker to scale your risk assessment capabilities. The early-warning signs provided by machine learning can make the difference between catching risks and having to deal with them after the fact.
Trading and Investment Management
Machine learning algorithms can analyze publicly available data — market trends, news and social media data — as well as privately held data to identify investment opportunities and make predictions about asset prices. These algorithms can also be used to manage portfolios and adjust investments based on market conditions.
Regulatory Compliance
Artificial intelligence and machine learning techniques are being used to ensure regulatory compliance. Financial institutions are heavily regulated, and failure to comply can result in civil and even criminal penalties, not to mention reputational damage. AI-powered systems can use machine learning algorithms to flag potential compliance violations and generate reports for regulatory agencies in a timely fashion.
Credit Scoring
Credit scores have long been calculated using a limited set of data points, such as payment history, outstanding debt and length of credit history. These criteria aren’t necessarily a complete or accurate representation of the consumer’s credit-worthiness. Machine learning can analyze a wider range of data points, including online behavior, to provide a more accurate assessment and reduce the likelihood of bias. The result is fairer lending decisions and more opportunities for borrowers who are overlooked or even discriminated against by traditional credit scoring models.
Data Security
Cybersecurity is incredibly complex for companies to manage, especially when they have sensitive financial data. Machine learning algorithms can analyze patterns in user behavior to identify potential security threats, such as suspicious logins or unusual activity. As part of a larger cybersecurity and data protection program, machine learning can help you prevent security lapses instead of solely responding to them.
Amazon Macie is an example of a powerful security tool that uses machine learning to automatically discover, classify and protect sensitive data stored in AWS. This service applies appropriate security controls to data, such as encrypting or restricting access, reducing the risk of breaches and protecting your data.
Algorithmic Trading
Algorithmic trading uses computer programs to execute trades based on predetermined rules and algorithms. Machine learning can be used to analyze market data, identify trends and contribute to more effective and efficient trading strategies.
An emerging trend in the industry is moving these types of solutions to the cloud. As exchanges and data providers migrate, there’s a greater incentive for financial firms to follow suit. This allows them to be closer, leverage the many benefits of the cloud and obtain low latencies, which is essential for high-frequency trading. These solutions can be developed and deployed using tools such as Amazon SageMaker and AWS Data Exchange. If your firm is looking to take this step, you should consider a migration readiness assessment.
Marketing
Marketing might not be the first thing you think of with machine learning. But your firm can improve its marketing efforts by learning from algorithms that analyze customer data, behavior and preferences. Strong machine learning practices help your firm develop personalized marketing campaigns and product recommendations.
Other artificial intelligence applications can help you customize campaigns even further. For example, NLP can be used to analyze customer feedback from social media, online reviews and other interactions. This data can be used to identify customer preferences or areas where they may be dissatisfied, allowing you to tailor messaging to better meet their needs.
Customer Experience
Artificial intelligence and machine learning can improve your overall customer experience. For example, chatbots and virtual assistants can provide quick and efficient customer service. Personalized recommendations move customers closer to purchasing decisions because they feel a connection to what’s being offered. Machine learning algorithms can also be used to predict customer needs and offer proactive solutions.
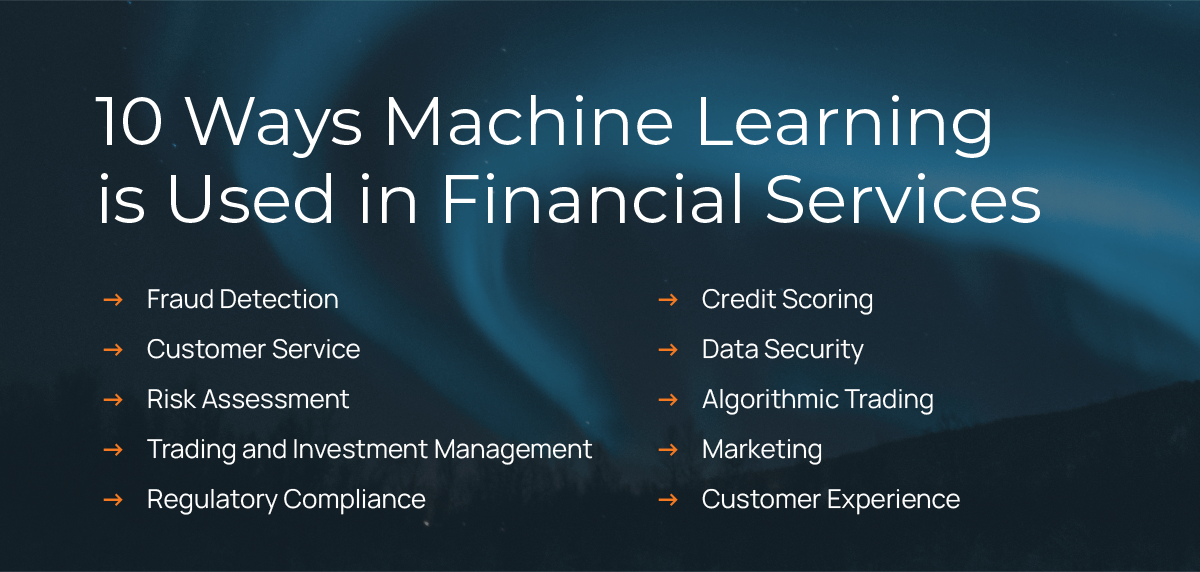
What’s Next for Financial Services Firms?
It's clear that machine learning is only growing in importance. New applications of machine learning in finance will continue to emerge. You can expect machine learning algorithms of the future to streamline operations, automate tasks, improve business decision-making and continue to personalize the customer experience.
One of the biggest challenges the industry will continue to face is the need for skilled talent to serve as data scientists, analysts and other vital roles. Be prepared to fight for machine learning talent or find a reliable third party that can get you the resources you need
The key to successfully leveraging artificial intelligence and machine learning in financial services is to look for ways to incorporate it into your existing business processes and workflows. Identify the specific pain points and inefficiencies in your operations, and explore how machine learning can help you solve these problems.
Learn how you can advance your strategy and leverage the cloud to successfully launch your projects.
FAQ
How do regulations and compliance issues affect the implementation of machine learning models in financial services, especially in different regions or countries with varying legal frameworks?
Implementing machine learning (ML) models within the financial services sector is heavily influenced by the regulatory and compliance landscape, which can vary significantly across different regions and countries. Financial institutions must navigate a complex web of laws and regulations to protect consumers, ensure market stability, and prevent financial crimes. These regulations often require rigorous validation and documentation of ML models, transparency in decision-making processes, and the ability to explain model decisions to regulators and customers. To address these challenges, financial services firms are investing in robust compliance frameworks that include model risk management practices, ensuring that their ML applications meet both internal standards and external regulatory requirements. This approach helps in mitigating risks associated with non-compliance, such as financial penalties and reputational damage, while leveraging the benefits of ML for innovation and competitive advantage.
What are the ethical considerations and potential biases in machine learning applications in financial services, and how can these be mitigated to ensure fair and equitable treatment of all customers?
Ethical considerations and potential bias in ML applications are critical concerns within the financial services industry. The data used to train ML models can reflect historical biases, leading to unfair or discriminatory outcomes, particularly in sensitive areas such as credit scoring, loan approvals, and insurance underwriting. Financial institutions are adopting ethical AI frameworks that emphasize fairness, accountability, and transparency to mitigate these risks. These frameworks guide the development and deployment of ML models, ensuring that they are designed and monitored to prevent bias and promote equitable treatment of all customers. Efforts include diverse data collection, regular bias audits, and the implementation of fairness metrics to evaluate and adjust models accordingly. By prioritizing ethical considerations, financial services firms aim to build trust with their customers and stakeholders, ensuring that ML technologies are used in a manner that aligns with societal values and norms.
Can machine learning models in financial services be transparent and explainable to customers, especially when used for critical decisions like loan approval or fraud detection, to build trust and understanding among users?
Transparency and explainability in ML models are essential for building trust and understanding among users, especially when these models inform critical financial decisions like loan approvals or fraud detection. Financial services firms are increasingly focused on developing explainable AI (XAI) that allows customers and regulators to understand how model decisions are made. This involves techniques providing insights into the model's reasoning, highlighting the factors influencing a particular decision. Explainable models help demystify ML processes, making them more accessible to non-technical stakeholders. Additionally, they facilitate compliance with regulations that require transparency in automated decision-making, such as the European Union's General Data Protection Regulation (GDPR), which includes rights to explanation for individuals affected by automated decisions. By investing in XAI, financial institutions enhance customer trust and ensure that their use of ML aligns with ethical standards and regulatory expectations, fostering a responsible approach to innovation in financial services.
Author Spotlight:
Ryan Ries
Keep Up To Date With AWS News
Stay up to date with the latest AWS services, latest architecture, cloud-native solutions and more.