Blog
What Is AWS Glue? Examples and How to Use It
In the era of big data, companies face the challenge of consolidating data from multiple sources into a single system. That’s why so many enterprises are turning to AWS Glue. Examples of its effectiveness include its speed, ease of use and cost compared with traditional extract, transform, load (ETL) processes.
AWS Glue makes it easier to move S3 data and many other data sources into a data warehouse for analysis. It can handle complicated, time-consuming work, especially when you know how to use the system effectively. AWS Glue does have a learning curve, however. If you don’t avoid common mistakes, you’ll produce incorrect data and contribute to misinformed decision-making.
Learn more about AWS Glue, when to use it and how to maximize its potential while avoiding easy mistakes.
What Is AWS Glue?
AWS Glue is a fully managed, serverless data integration service that makes it easy to prepare and load data for analytics. It provides a flexible, cost-effective way to move and transform data between on-premises and cloud-based data stores.
You can use AWS Glue to build sophisticated cloud-based data lakes, or centralized data repositories. The service also automates the time-consuming tasks of data discovery, mapping and job scheduling, freeing up your organization to focus on the data.
AWS Glue is a collection of features, including:
- AWS Glue Data Catalog: Allows you to catalog data assets and make them available across all AWS analytics services.
- AWS Glue crawlers: Perform data discovery on data sources.
- AWS Glue jobs: Execute the ETL in your pipeline in either Python or Scala. Python scripts use an extension of the PySpark Python dialect for ETL jobs.
Users can also interact with AWS Glue via a graphical user interface by using services including AWS Glue DataBrew and AWS Glue Studio. Taking this step makes the service more accessible for complex tasks, such as data processing, without advanced technical skills such as code generation or editing.
With AWS Glue, you only pay for the resources you use. There’s no minimum fee and no upfront commitment. The service is one of several tools offered by AWS for ETL processes, and can be used in addition to other tools such as Amazon EMR Serverless.
What Are the Benefits of AWS Glue?
AWS Glue is a cost-effective and easy-to-use ETL service that can process structured and unstructured data. The time spent developing an ETL solution can be reduced from months down to as little as minutes. Here are the key benefits of using AWS Glue.
Automated Data Discovery
Data discovery, which is the process of identifying and categorizing data, can be time-consuming, especially with large amounts of data spread across multiple sources. AWS Glue helps automate this process by crawling your data sources and identifying their schema.
Simple Data Cleansing
Cleaning up inaccurate or incomplete data is critical, especially before you load data into a new system or prepare it for analysis. AWS Glue can help you clean up data with its built-in transforms.
Easy ETL
ETL is a process many data-driven companies use to consolidate data from multiple sources. This process can be time-consuming and expensive, but AWS Glue can help you automate it. With AWS Glue, you only need to define the ETL job once. From there, the system extracts the data from the source, completes the data transformation process and automatically generates the desired format at the destination.
Help Preparing Data
Data preparation is essential for any analytics project. Raw data typically needs cleaning, normalization and aggregation before being analyzed. AWS Glue helps you prepare your data with its built-in transforms.
Data Migration Support
AWS Glue can migrate on-premises data stores to Amazon S3. This is often done as part of a larger cloud migration strategy. With AWS Glue, you can migrate your data without having to rewrite existing ETL jobs.
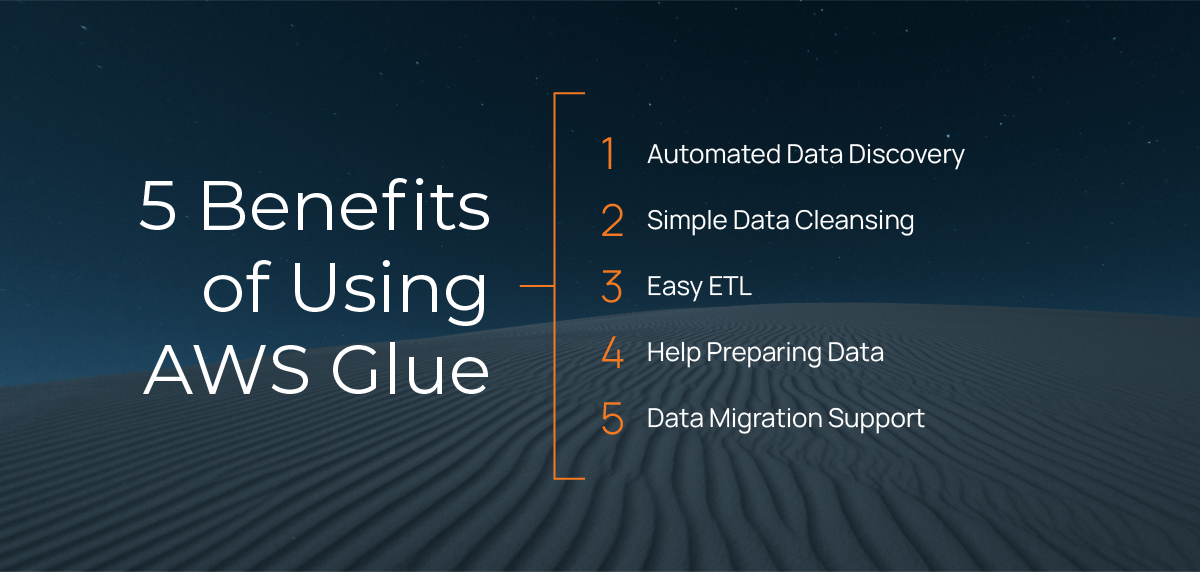
What Are the Challenges of Using AWS Glue?
AWS Glue offers time-saving benefits for your operational ETL pipeline, but it’s important to be aware of the common mistakes that even experienced users make. These mistakes often stem from not understanding the service’s nuances.
Learn more about three key places where errors most commonly occur.
Source Data
Source data is a crucial aspect of a data pipeline and needs to be properly managed. To ensure a smooth pipeline, review the common mistakes, and invest time in verifying data quality.
One common mistake is using incorrect file formats. File formats play a critical role in the pipeline and must be splittable to fit into Spark executor memory. Common splittable formats include CSV, Parquet, and ORC, while non-splittable formats include XML and JSON. While AWS Glue automatically supports file splitting, consider using columnar formats like Parquet for better performance.
Another mistake is when data quality isn’t verified. This leads to AWS Glue crawlers misinterpreting data and other unexpected behaviors. Use a service like AWS Glue DataBrew to explore the dataset. Incorporate data cleansing into the ETL processes. If needed, use Amazon Athena’s pseudo-column "$path" to locate fields in the underlying data.
Another common mistake is operating over small files, which causes performance issues. During the creation of the dynamic frame, or DataFrame, the Spark driver will create a list in memory of all the files to be included. Until that list is completed, the object won’t be created. Select the "useS3ListImplementation" feature in AWS Glue to enable lazy loading of S3 objects and reduce memory pressure on the driver. The grouping option in AWS Glue can also help by consolidating files for group-level reads, which reduces the number of tasks.
AWS Glue Crawler
AWS Glue Crawler is a valuable tool for companies that want to offload the task of determining and defining the schema of structured and semi-structured datasets.
Getting the crawler right starts with the right configuration and correctly defining the data catalog. Crawlers often come before or after ETL jobs, meaning the length of time to crawl a dataset affects the overall time to complete the pipeline. Meanwhile, the dataset’s metadata, as determined by the crawler, is used across most of the AWS analytics services.
A common mistake is failing to crawl a subset of data, leading to long-running crawlers. To overcome this, enable incremental crawls using the option “Crawl new folders only.” Alternatively, use include and exclude patterns to identify specific data that needs to be crawled.
For large datasets, you can use multiple smaller crawlers, each pointing to a subset of data, instead of one large crawler. Another solution is to use data sampling for databases and “sample size” for S3 buckets. With this configuration, only a sample of data will be crawled, reducing the overall time.
Another frequent issue is failing to optimally configure crawler discovery. The result is more tables than you expected. To mitigate this, select the option “Create a single schema for each S3 path.” The crawler will consider data compatibility and schema similarity, grouping the data into a single table if both requirements are met.
Sometimes, this fix doesn’t work. If the crawler continues creating more tables than expected, look at the underlying data to determine the cause.
AWS Glue Jobs
AWS Glue jobs are the heart of the AWS Glue service, as they execute the ETL. The AWS Glue console and services such as AWS Glue Studio will generate scripts for this task. However, many customers opt to write their own Spark code, which introduces a higher risk of error.
A common mistake is failing to use DynamicFrames correctly. DynamicFrames are specific to AWS Glue. Compared with traditional Spark DataFrames, they are an improvement by being self-describing and better able to handle unexpected values. However, some operations still require DataFrames, which can lead to costly conversions. The goal should be to start with a DynamicFrame, convert to a DataFrame only if necessary, and finish with a DynamicFrame.
Another common mistake is incorrect usage of job bookmarks. Job bookmarks allow tracking of processed data in each run of an ETL job from sources including S3 or JDBC. Bookmarks enable you to “rewind” a job to reprocess a subset of data or reset the bookmark for backfilling. If critical components are missing in custom-developed jobs, bookmarks won’t function correctly. Consider using the ETL script generated by AWS Glue as a reference.
Another mistake with AWS Glue Jobs is failing to partition data correctly. By default, AWS Glue-generated scripts don’t partition when writing job output. Take advantage of pushdown predicates to filter partitions without having to list and read all the files in the dataset. Pushdown predicates are evaluated before S3 Lister, making them a valuable tool in improving performance.
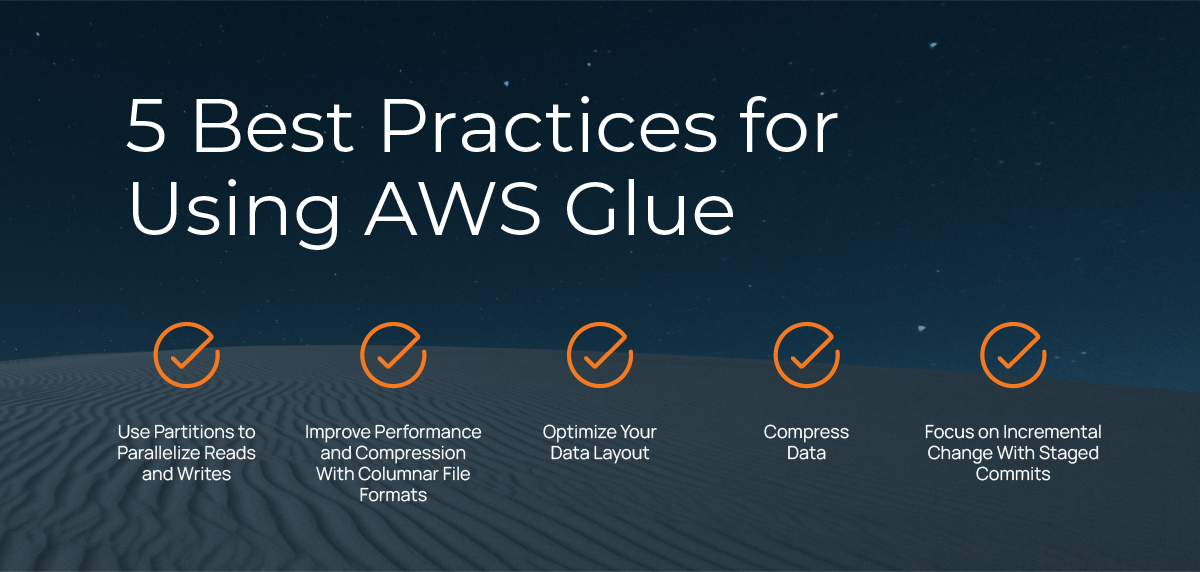
What Are Best Practices for Using AWS Glue?
Just as there are best practices for avoiding mistakes with AWS Glue, there are also common tips for getting the most out of this service. Here are five of them.
Use Partitions to Parallelize Reads and Writes
Partitions are a key part of how AWS Glue processes data. By dividing up your data into partitions, you can parallelize reads and writes, which improves performance and reduces costs. When creating partitions, consider the size of your data, the required partitions and the system load.
Improve Performance and Compression With Columnar File Formats
Columnar file formats are a type of file format that's optimized for column-oriented data stores. These formats are often used in data warehouses and analytics applications because they provide superior performance and compression. When using columnar file formats with AWS Glue, consider the size of your data, the number of columns and the available compression codecs.
Optimize Your Data Layout
Data layout can have a big impact on performance. When designing a layout, consider the size of your data, the number of columns, the type of storage and whether you need to support multiple versions of your data.
Compress Data
By compressing your data, you can improve performance and save on storage costs. There are many compression codecs available, so look for those supported by AWS Glue and that work well with your particular dataset.
Focus on Incremental Change With Staged Commits
When committing changes to Amazon S3, use staged commits rather than large commits. Staged commits allow you to commit changes in small batches, reducing the risk of failures and rollbacks. This approach is especially helpful if you’re new to AWS Glue.
Leverage AWS Glue Auto Scaling
Auto Scaling allows you to scale AWS Glue Spark jobs automatically based on the dynamically calculated requirements during job runs, helping improve efficiency and performance while reducing costs. This is particularly useful when dealing with large and unpredictable amounts of data in the cloud.
This means you no longer need to manually plan for capacity ahead of time or experiment with the data to determine how much capacity is required. Instead, you simply specify the maximum number of workers required and AWS Glue dynamically allocates resources based on workload requirements during job runs, adding more worker nodes to the cluster in near-real time when Spark requests more executors.
Use Interactive Sessions for Jupyter
Interactive sessions provide a highly-scalable, serverless Spark backend to Jupyter notebooks and Jupyter-based IDEs, allowing for on-demand and efficient interactive job development with AWS Glue. By using interactive sessions, there are no clusters to provision or manage, no idle clusters to pay for, and no up-front configuration required, making it an easy and cost-effective option. Plus, resource contention for the same development environment is eliminated, and the exact same serverless Spark runtime and platform as AWS Glue ETL jobs are used.
Using interactive sessions for Jupyter can significantly improve the efficiency of your AWS Glue development and help you save on costs.
Finding Success With AWS Glue
AWS Glue is a powerful service with defined best practices to guide you. It can be one of the most useful, versatile and robust tools in your AWS data ecosystem. With its serverless architecture, automatic scaling and data discovery and catalog capabilities, AWS Glue offers an efficient and cost-effective solution for your data integration needs.
While AWS Glue has tremendous potential, it’s only as good as the user. Make sure you’re avoiding rookie mistakes, and learn from AWS Glue examples so you can maximize its potential within your organization.
By partnering with an AWS Premier Consulting Partner like Mission Cloud, you can simplify your data integrations and easily access critical business analytics. Learn how to find support and gain confidence in your data strategy by implementing a serverless, cost-effective solution like AWS Glue.
FAQ
How does AWS Glue handle real-time data processing?
AWS Glue is primarily designed for batch processing, yet it does offer capabilities that extend into the realm of real-time data processing. For scenarios requiring immediate data handling, AWS Glue can be complemented with other AWS services like Amazon Kinesis. This combination allows for the ingestion and processing of streaming data in real-time. Through triggers and job scheduling, AWS Glue can respond to new data as it arrives, ensuring that analytics and data storage systems are continuously updated. This approach enables businesses to achieve a near real-time data processing pipeline, leveraging AWS Glue's powerful ETL capabilities alongside streaming data services for comprehensive data integration and analytics solutions.
Can AWS Glue integrate with on-premises databases, or is it limited to AWS services?
Regarding integrating on-premises databases, AWS Glue offers a versatile solution that is not limited to cloud-based sources and targets. Organizations can establish a secure and reliable connection between their on-premises databases and AWS Glue through the AWS Direct Connect service. This setup facilitates the direct transfer of data, allowing AWS Glue to perform ETL operations on data residing in on-premises databases as seamlessly as it does with data in the cloud. This capability is particularly beneficial for businesses operating in a hybrid cloud environment, as it provides a unified platform for data integration and transformation across diverse data sources, ensuring flexibility and scalability in data management strategies.
What are the best practices for optimizing AWS Glue ETL job performance?
Optimizing the performance of AWS Glue ETL jobs involves a combination of adjusting resource allocation, monitoring job metrics, and employing efficient coding practices. It's essential to tailor the resources assigned to each job to enhance job performance based on the volume and complexity of the data being processed. Monitoring tools provided by AWS Glue offers insights into job execution metrics, enabling the identification of bottlenecks and areas for improvement. Additionally, optimizing the code of ETL scripts by minimizing the amount of data shuffled across the network and leveraging pushdown predicates can significantly reduce job runtimes and costs. Employing partitioning strategies and choosing the appropriate data formats are also crucial steps in improving the efficiency of data processing tasks. Together, these practices ensure that AWS Glue ETL jobs are optimized for both performance and cost-effectiveness, supporting scalable and efficient data integration workflows.
Author Spotlight:
Ryan Ries
Keep Up To Date With AWS News
Stay up to date with the latest AWS services, latest architecture, cloud-native solutions and more.